Quantitative evaluation of CT scan images to determinate the prognosis of COVID-19 patient using deep learning
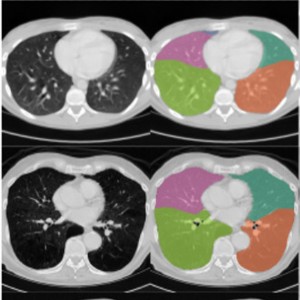
Accepted: 13 July 2023
HTML: 2
All claims expressed in this article are solely those of the authors and do not necessarily represent those of their affiliated organizations, or those of the publisher, the editors and the reviewers. Any product that may be evaluated in this article or claim that may be made by its manufacturer is not guaranteed or endorsed by the publisher.
The purpose of this research is to evaluate the accuracy of AI-assisted quantification in comparison to conventional CT parameters reviewed by a radiologist in predicting the severity, progression, and clinical outcome of disease. The current study is a cross-sectional study that was conducted on patients with the diagnosis of COVID-19 and underwent a pulmonary CT scan between August 23th, 2021 to December 21th, 2022. The initial CT scan on admission was used for imaging analysis. The presence of ground glass opacity (GGO), and consolidation were visually evaluated. CT severity score was calculated according to a semi-quantitative method. In addition, AI based quantification of GGO and consolidation volume were also performed. 291 patients (mean age: 64.7 ± 7; 129 males) were included. GGO + consolidation was more frequently revealed in progress-to-severe group whereas pure GGO was more likely to be found in non-severe group. Compared to non-severe group, patients in progress-to-severe group had larger GGO volume percentage (40.6%± 11.9%versus 21.7%± 8.8%, p ˂0.001) as well as consolidation volume percentage (4.8% ± 2% versus 1.9% ± 1%, p < 0.001). Among imaging parameters, consolidation volume percentage and the largest area under curve (AUC) in discriminating non-severe from progress-to-severe group (AUC = 0.91, p < 0.001). According to multivariate regression, consolidation volume was the strongest predictor for disease progression. In conclusion, the consolidation volume measured on the initial chest CT was the most accurate predictor of disease progression, and a larger consolidation volume was associated with a poor clinical outcome. In patients with COVID-19, AI-assisted lesion quantification was useful for risk stratification and prognosis evaluation.
Ciotti M, Ciccozzi M, Terrinoni A, Jiang WC, Wang CB, Bernardini S. The COVID-19 pandemic. Crit Rev Clin Lab Sci. 2020 Sep;57(6):365-388. Epub 2020 Jul 9. PMID: 32645276. DOI: https://doi.org/10.1080/10408363.2020.1783198
Msemburi W, Karlinsky A, Knutson V, Aleshin-Guendel S, Chatterji S, Wakefield J. The WHO estimates of excess mortality associated with the COVID-19 pandemic. Nature. 2023 Jan;613(7942):130-137. Epub 2022 Dec 14. PMID: 36517599; PMCID: PMC9812776. DOI: https://doi.org/10.1038/s41586-022-05522-2
Kang J, Kang J, Seo WJ, Park SH, Kang HK, Park HK, Hyun J, Song JE, Kwak YG, Kim KH, Kim YS, Lee SS, Koo HK. Prediction models for respiratory outcomes in patients with COVID-19: integration of quantitative computed tomography parameters, demographics, and laboratory features. J Thorac Dis. 2023 Mar 31;15(3):1506-1516. Epub 2023 Mar 9. PMID: 37065603; PMCID: PMC10089866. DOI: https://doi.org/10.21037/jtd-22-1076
Vandenberg O, Martiny D, Rochas O, van Belkum A, Kozlakidis Z. Considerations for diagnostic COVID-19 tests. Nat Rev Microbiol. 2021 Mar;19(3):171-183. Epub 2020 Oct 14. PMID: 33057203; PMCID: PMC7556561. DOI: https://doi.org/10.1038/s41579-020-00461-z
Jafari R, Cegolon L, Jafari A, Kashaki M, Otoukesh B, Ghahderijani BH, Izadi M, Saadat SH, Einollahi B, Javanbakht M. Large saddle pulmonary embolism in a woman infected by COVID-19 pneumonia. Eur Heart J. 2020 Jun 7;41(22):2133. PMID: 32374381; PMCID: PMC7239188. DOI: https://doi.org/10.1093/eurheartj/ehaa402
Long C, Xu H, Shen Q, Zhang X, Fan B, Wang C, Zeng B, Li Z, Li X, Li H. Diagnosis of the Coronavirus disease (COVID-19): rRT-PCR or CT? Eur J Radiol. 2020 May;126:108961. Epub 2020 Mar 25. PMID: 32229322; PMCID: PMC7102545. DOI: https://doi.org/10.1016/j.ejrad.2020.108961
Vaishya R, Javaid M, Khan IH, Haleem A. Artificial Intelligence (AI) applications for COVID-19 pandemic. Diabetes Metab Syndr. 2020 Jul-Aug;14(4):337-339. Epub 2020 Apr 14. PMID: 32305024; PMCID: PMC7195043. DOI: https://doi.org/10.1016/j.dsx.2020.04.012
Chen J, See KC. Artificial Intelligence for COVID-19: Rapid Review. J Med Internet Res. 2020 Oct 27;22(10):e21476. PMID: 32946413; PMCID: PMC7595751. DOI: https://doi.org/10.2196/21476
Khan M, Mehran MT, Haq ZU, Ullah Z, Naqvi SR, Ihsan M, Abbass H. Applications of artificial intelligence in COVID-19 pandemic: A comprehensive review. Expert Syst Appl. 2021 Dec 15;185:115695. Epub 2021 Aug 4. PMID: 34400854; PMCID: PMC8359727. DOI: https://doi.org/10.1016/j.eswa.2021.115695
Yang R, Li X, Liu H, Zhen Y, Zhang X, Xiong Q, Luo Y, Gao C, Zeng W. Chest CT Severity Score: An Imaging Tool for Assessing Severe COVID-19. Radiol Cardiothorac Imaging. 2020 Mar 30;2(2):e200047. PMID: 33778560; PMCID: PMC7233443. DOI: https://doi.org/10.1148/ryct.2020200047
Pan F, Ye T, Sun P, Gui S, Liang B, Li L, Zheng D, Wang J, Hesketh RL, Yang L, Zheng C. Time Course of Lung Changes at Chest CT during Recovery from Coronavirus Disease 2019 (COVID-19). Radiology. 2020 Jun;295(3):715-721. Epub 2020 Feb 13. PMID: 32053470; PMCID: PMC7233367. DOI: https://doi.org/10.1148/radiol.2020200370
Ronneberger O, Fischer P, Brox T, editors. U-net: Convolutional networks for biomedical image segmentation. Medical Image Computing and Computer-Assisted Intervention–MICCAI 2015: 18th International Conference, Munich, Germany, October 5-9, 2015, Proceedings, Part III 18; 2015: Springer.
Zhou X, Takayama R, Wang S, Hara T, Fujita H. Deep learning of the sectional appearances of 3D CT images for anatomical structure segmentation based on an FCN voting method. Med Phys. 2017 Oct;44(10):5221-5233. Epub 2017 Aug 31. PMID: 28730602. DOI: https://doi.org/10.1002/mp.12480
Isensee F, Petersen J, Klein A, Zimmerer D, Jaeger PF, Kohl S. Nnu-net: Self-adapting framework for u-net-based medical image segmentation. arXiv preprint arXiv:180910486. 2018. DOI: https://doi.org/10.1007/978-3-658-25326-4_7
Hofmanninger J, Prayer F, Pan J, Röhrich S, Prosch H, Langs G. Automatic lung segmentation in routine imaging is primarily a data diversity problem, not a methodology problem. Eur Radiol Exp. 2020 Aug 20;4(1):50. PMID: 32814998; PMCID: PMC7438418. DOI: https://doi.org/10.1186/s41747-020-00173-2
Naudé W. Artificial intelligence vs COVID-19: limitations, constraints and pitfalls. AI Soc. 2020;35(3):761-765. Epub 2020 Apr 28. PMID: 32346223; PMCID: PMC7186767. DOI: https://doi.org/10.1007/s00146-020-00978-0
Hope MD, Raptis CA, Shah A, Hammer MM, Henry TS; six signatories. A role for CT in COVID-19? What data really tell us so far. Lancet. 2020 Apr 11;395(10231):1189-1190. Epub 2020 Mar 27. PMID: 32224299; PMCID: PMC7195087. DOI: https://doi.org/10.1016/S0140-6736(20)30728-5
Ai T, Yang Z, Hou H, Zhan C, Chen C, Lv W, Tao Q, Sun Z, Xia L. Correlation of Chest CT and RT-PCR Testing for Coronavirus Disease 2019 (COVID-19) in China: A Report of 1014 Cases. Radiology. 2020 Aug;296(2):E32-E40. Epub 2020 Feb 26. PMID: 32101510; PMCID: PMC7233399. DOI: https://doi.org/10.1148/radiol.2020200642
Zhou C, Chan HP, Chughtai A, Patel S, Kuriakose J, Hadjiiski LM, Wei J, Kazerooni EA. Variabilities in Reference Standard by Radiologists and Performance Assessment in Detection of Pulmonary Embolism in CT Pulmonary Angiography. J Digit Imaging. 2019 Dec;32(6):1089-1096. PMID: 31073815; PMCID: PMC6841909. DOI: https://doi.org/10.1007/s10278-019-00228-w
Richardson S, Hirsch JS, Narasimhan M, Crawford JM, McGinn T, Davidson KW; the Northwell COVID-19 Research Consortium; Barnaby DP, Becker LB, Chelico JD, Cohen SL, Cookingham J, Coppa K, Diefenbach MA, Dominello AJ, Duer-Hefele J, Falzon L, Gitlin J, Hajizadeh N, Harvin TG, Hirschwerk DA, Kim EJ, Kozel ZM, Marrast LM, Mogavero JN, Osorio GA, Qiu M, Zanos TP. Presenting Characteristics, Comorbidities, and Outcomes Among 5700 Patients Hospitalized With COVID-19 in the New York City Area. JAMA. 2020 May 26;323(20):2052-2059. Erratum in: JAMA. 2020 May 26;323(20):2098. PMID: 32320003; PMCID: PMC7177629. DOI: https://doi.org/10.1001/jama.2020.6775
Salman FM, Abu-Naser SS, Alajrami E, Abu-Nasser BS, Alashqar BA. Covid-19 detection using artificial intelligence. International Journal of Academic Engineering Research (IJAER). ISSN: 2643-9085. Vol. 4, Issue 3, March – 2020, Pages: 18-252020.
Kim CK, Choi JW, Jiao Z, Wang D, Wu J, Yi TY, Halsey KC, Eweje F, Tran TML, Liu C, Wang R, Sollee J, Hsieh C, Chang K, Yang FX, Singh R, Ou JL, Huang RY, Feng C, Feldman MD, Liu T, Gong JS, Lu S, Eickhoff C, Feng X, Kamel I, Sebro R, Atalay MK, Healey T, Fan Y, Liao WH, Wang J, Bai HX. An automated COVID-19 triage pipeline using artificial intelligence based on chest radiographs and clinical data. NPJ Digit Med. 2022 Jan 14;5(1):5. PMID: 35031687; PMCID: PMC8760275. DOI: https://doi.org/10.1038/s41746-021-00546-w
Soda P, D'Amico NC, Tessadori J, Valbusa G, Guarrasi V, Bortolotto C, Akbar MU, Sicilia R, Cordelli E, Fazzini D, Cellina M, Oliva G, Callea G, Panella S, Cariati M, Cozzi D, Miele V, Stellato E, Carrafiello G, Castorani G, Simeone A, Preda L, Iannello G, Del Bue A, Tedoldi F, Alí M, Sona D, Papa S. AIforCOVID: Predicting the clinical outcomes in patients with COVID-19 applying AI to chest-X-rays. An Italian multicentre study. Med Image Anal. 2021 Dec;74:102216. Epub 2021 Aug 28. PMID: 34492574; PMCID: PMC8401374. DOI: https://doi.org/10.1016/j.media.2021.102216
Li Y, Shang K, Bian W, He L, Fan Y, Ren T, Zhang J. Prediction of disease progression in patients with COVID-19 by artificial intelligence assisted lesion quantification. Sci Rep. 2020 Dec 16;10(1):22083. PMID: 33328512; PMCID: PMC7745019. DOI: https://doi.org/10.1038/s41598-020-79097-1
Li K, Wu J, Wu F, Guo D, Chen L, Fang Z, Li C. The Clinical and Chest CT Features Associated With Severe and Critical COVID-19 Pneumonia. Invest Radiol. 2020 Jun;55(6):327-331. PMID: 32118615; PMCID: PMC7147273. DOI: https://doi.org/10.1097/RLI.0000000000000672
Lyu P, Liu X, Zhang R, Shi L, Gao J. The Performance of Chest CT in Evaluating the Clinical Severity of COVID-19 Pneumonia: Identifying Critical Cases Based on CT Characteristics. Invest Radiol. 2020 Jul;55(7):412-421. PMID: 32304402; PMCID: PMC7173027. DOI: https://doi.org/10.1097/RLI.0000000000000689
Barton LM, Duval EJ, Stroberg E, Ghosh S, Mukhopadhyay S. COVID-19 Autopsies, Oklahoma, USA. Am J Clin Pathol. 2020 May 5;153(6):725-733. Erratum in: Am J Clin Pathol. 2020 May 5;153(6):852. PMID: 32275742; PMCID: PMC7184436. DOI: https://doi.org/10.1093/ajcp/aqaa062
Koo HJ, Choi SH, Sung H, Choe J, Do KH. RadioGraphics Update: Radiographic and CT Features of Viral Pneumonia. Radiographics. 2020 Jul-Aug;40(4):E8-E15. Epub 2020 Jun 5. PMID: 32501740; PMCID: PMC7336757. DOI: https://doi.org/10.1148/rg.2020200097
Ooi GC, Khong PL, Müller NL, Yiu WC, Zhou LJ, Ho JC, Lam B, Nicolaou S, Tsang KW. Severe acute respiratory syndrome: temporal lung changes at thin-section CT in 30 patients. Radiology. 2004 Mar;230(3):836-44. PMID: 14990845. DOI: https://doi.org/10.1148/radiol.2303030853
Ren HW, Wu Y, Dong JH, An WM, Yan T, Liu Y, Liu CC. Analysis of clinical features and imaging signs of COVID-19 with the assistance of artificial intelligence. Eur Rev Med Pharmacol Sci. 2020 Aug;24(15):8210-8218. PMID: 32767351.
Meiler S, Schaible J, Poschenrieder F, Scharf G, Zeman F, Rennert J, Pregler B, Kleine H, Stroszczynski C, Zorger N, Hamer OW. Can CT performed in the early disease phase predict outcome of patients with COVID 19 pneumonia? Analysis of a cohort of 64 patients from Germany. Eur J Radiol. 2020 Oct;131:109256. Epub 2020 Aug 28. PMID: 32919265; PMCID: PMC7452844. DOI: https://doi.org/10.1016/j.ejrad.2020.109256
Honarmand A, Sheybani F, Aflatoonian E, Saberinia A. COVID-19 patients at referral to hospital during the first peak of disease: Common clinical findings including myalgia and fatigue. Eur J Transl Myol. 2022 Aug 12;32(3). PMID: 36036352. DOI: https://doi.org/10.4081/ejtm.2022.10731
Copyright (c) 2023 the Author(s)

This work is licensed under a Creative Commons Attribution-NonCommercial 4.0 International License.
PAGEPress has chosen to apply the Creative Commons Attribution NonCommercial 4.0 International License (CC BY-NC 4.0) to all manuscripts to be published.